Nvidia’s shrinking market cap could signal a shift in the generative AI developer’s market.
The use of Google’s chips by Apple for AI training is an interesting development. Apple’s move to utilize Google’s hardware, specifically the Tensor Processing Units (TPUs), suggests that they are exploring different avenues for their AI and machine learning needs.
Nvidia, a major player in the GPU market, remains a significant force in AI and machine learning. Nvidia’s GPUs are widely used for their performance in training and inference tasks due to their parallel processing capabilities. However, the landscape of AI hardware is evolving, and companies like Google and Apple are investing in custom solutions like TPUs and Apple’s own silicon to meet their specific needs. train
Here’s how this affects Nvidia:
- Competition and Innovation: The adoption of different chips for AI training could drive Nvidia to innovate further. Nvidia might accelerate development of their hardware or software to stay competitive.
- Market Segmentation: Nvidia’s GPUs are still highly regarded, especially in sectors where their architecture excels. Apple’s choice to use Google’s chips doesn’t necessarily mean a decline in Nvidia’s overall market position, but rather reflects the diversity of solutions available.
- Strategic Partnerships: Nvidia’s relationships with various tech giants and cloud providers might help mitigate the impact. They could focus on strengthening their partnerships and expanding their influence in AI and other computing areas. train
Overall, while Apple’s use of Google’s chips might represent a shift in the AI hardware landscape, Nvidia’s established position and ongoing innovations keep it a key player in the field.
1. Apple’s AI Strategy
Apple’s decision to use Google’s Tensor Processing Units (TPUs) reflects a strategic move to leverage advanced AI hardware that might offer specific advantages. TPUs are designed for high-performance machine learning tasks and are optimized for TensorFlow, Google’s machine learning framework. By using TPUs, Apple could be aiming to enhance its AI capabilities efficiently, potentially improving performance or reducing costs.
2. Nvidia’s Position in AI Hardware
Nvidia has established itself as a leader in AI and machine learning through its Graphics Processing Units (GPUs), which are highly effective for parallel processing tasks. Their GPUs, like the A100 and H100, are widely used in data centers for training large AI models. Nvidia’s CUDA programming model and software ecosystem also contribute to their strong position in the market. train
3. Emerging AI Hardware Solutions
The landscape of AI hardware is becoming increasingly diverse:
- Google TPUs: Custom-built for TensorFlow, TPUs offer high performance for specific types of AI tasks.
- Apple Silicon: Apple is investing heavily in its own silicon, including the M1 and M2 chips, which have specialized AI processing capabilities through their Neural Engine.
- Other Competitors: Companies like Intel, AMD, and startups are also developing specialized AI hardware, including custom accelerators and chips designed to meet specific performance needs. train
4. Impact on Nvidia
- Innovation and Competition: Nvidia may need to accelerate innovation in its hardware and software to maintain its competitive edge. This could involve developing new generations of GPUs, expanding their AI software stack, or exploring new architectures.
- Market Adaptation: Nvidia’s GPUs remain versatile and powerful, suitable for a wide range of applications beyond AI, such as gaming, simulation, and professional graphics. They might focus on these strengths while adapting to new trends in AI hardware.
- Partnerships and Ecosystems: Nvidia could strengthen its strategic partnerships with tech companies, cloud providers, and research institutions. They might also invest in new AI frameworks and software to complement their hardware offerings.
5. The Bigger Picture
- Diversification of AI Hardware: The trend towards using specialized hardware reflects a broader movement towards optimizing AI infrastructure for specific needs. This diversification could lead to more efficient and capable AI systems but also means that no single company will dominate the market completely.
- Customer Needs: Different companies have different requirements for AI training and inference. Some may prefer the flexibility and performance of Nvidia’s GPUs, while others might opt for the tailored capabilities of TPUs or custom silicon.
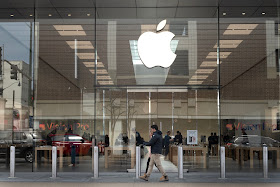
In summary, while Apple’s use of Google’s TPUs highlights a shift in AI hardware preferences, Nvidia’s strong position and ongoing innovation efforts help it remain a key player in the field. The evolving landscape will likely continue to see a mix of different technologies being used to meet diverse AI needs.
1. Apple’s Use of Google’s TPUs
TPUs (Tensor Processing Units):
- Purpose-built: Google’s TPUs are designed specifically for accelerating TensorFlow computations. They excel in handling large-scale matrix operations and deep learning models.
- Architecture: TPUs are optimized for high throughput and low latency in AI tasks, providing significant performance improvements over general-purpose processors.
Apple’s Strategy:
- Performance Gains: By using TPUs, Apple aims to enhance the performance of its machine learning models, particularly in tasks like natural language processing, computer vision, and other AI-driven features.
- Cost Efficiency: Leveraging TPUs could potentially lower costs associated with training large-scale AI models compared to using general-purpose GPUs.
2. Nvidia’s Position and Response
Nvidia GPUs:
- Architecture: Nvidia’s GPUs, such as the A100 and H100, are built for high-performance computing (HPC) and AI workloads. They feature CUDA cores, tensor cores, and support for large memory bandwidth, which are critical for training and deploying complex models.
- CUDA Ecosystem: Nvidia’s CUDA (Compute Unified Device Architecture) platform is a powerful parallel computing platform and application programming interface (API) model. It provides a robust software environment for developers to optimize their applications for Nvidia GPUs.
Potential Implications for Nvidia:
- Increased Competition: The adoption of TPUs and other specialized hardware by companies like Apple increases competition in the AI hardware market. Nvidia will need to differentiate itself by continuing to advance its GPU technology and software capabilities.
- Innovation: Nvidia may accelerate the development of new GPU architectures or hardware features to maintain its competitive edge. Innovations could include improved tensor core performance, enhanced memory management, and support for new AI frameworks.
- Partnerships and Collaborations: Nvidia may strengthen partnerships with cloud service providers, research institutions, and enterprise clients to leverage its GPU technology for a wide range of applications, including AI.
3. Broader AI Hardware Landscape
Emerging Technologies:
- Custom AI Chips: Companies like Apple and Google are investing in custom silicon to optimize performance for specific AI tasks. Apple’s Neural Engine and Google’s TPUs are examples of this trend.
- Other Companies: Intel’s Habana Labs and AMD’s Radeon Instinct are also developing AI accelerators. Intel’s Gaudi and Gaudi2 chips are designed for deep learning training, while AMD focuses on GPUs optimized for various AI workloads.
Market Trends:
- Diverse Requirements: Different AI tasks have varying requirements, leading to a diverse market for AI hardware. For example, some models may benefit from TPUs’ high throughput, while others might require the flexibility of GPUs.
- Adoption of New Architectures: As AI models become more complex, there is an increasing demand for specialized hardware that can efficiently handle these models. This trend could lead to more companies developing or adopting custom chips for AI tasks.
4. Nvidia’s Strategic Moves
Continued Investment in R&D:
- Hardware Development: Nvidia is likely to continue investing in research and development to enhance its GPU architecture, making it more efficient for AI tasks. Upcoming products and advancements could further solidify Nvidia’s position in the market.
Software Ecosystem:
- Expanding CUDA: Nvidia’s CUDA ecosystem is a critical part of its strategy. By continuously updating and expanding this ecosystem, Nvidia ensures that its hardware remains compatible with the latest AI frameworks and applications.
- AI Frameworks: Nvidia may also invest in optimizing or developing new AI frameworks and tools that work seamlessly with its GPUs.
Market Adaptation:
- Enterprise Solutions: Nvidia might focus on providing tailored solutions for various industries, including healthcare, finance, and autonomous vehicles. These solutions can leverage Nvidia’s GPU technology to address specific needs.
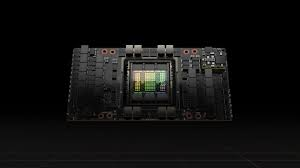
In summary, Apple’s use of Google’s TPUs highlights a growing trend towards specialized AI hardware. While this introduces increased competition for Nvidia, it also presents opportunities for Nvidia to innovate and adapt. Nvidia’s strong position in the GPU market, combined with its ongoing advancements in hardware and software, helps it remain a key player in the evolving AI landscape.
Advantages of Google’s TPUs
- Specialized for TensorFlow:
- Optimized Performance: TPUs are designed specifically for TensorFlow, Google’s open-source machine learning framework. This optimization can lead to significant performance improvements in training and inference tasks using TensorFlow.
- High Throughput: TPUs provide high throughput for matrix operations, which are common in deep learning algorithms. This can accelerate the training of complex models.
- Efficiency:
- Energy Efficiency: TPUs are engineered to perform AI computations with high energy efficiency, potentially lowering operational costs related to power and cooling.
- Cost Efficiency: For TensorFlow-based workloads, TPUs might offer a more cost-effective solution compared to GPUs, as they can achieve faster training times with potentially lower overall costs.
- Custom Hardware:
- Tailored Design: TPUs are purpose-built for AI tasks, leading to more efficient execution of certain operations compared to general-purpose hardware.
Disadvantages of Google’s TPUs
- Limited Flexibility:
- Framework Dependency: TPUs are optimized for TensorFlow, which might limit their effectiveness for models developed in other frameworks like PyTorch or MXNet. This could be a disadvantage for teams using multiple frameworks.
- Availability and Access:
- Cloud-Only Access: As of now, TPUs are primarily available through Google Cloud, which might limit their accessibility for organizations that prefer on-premises solutions or use other cloud providers.
- Niche Optimization:
- Not Universal: TPUs might not be as versatile as GPUs for a broader range of computing tasks outside of AI and machine learning.
Advantages of Nvidia’s GPUs
- Versatility:
- Broad Applicability: Nvidia GPUs are highly versatile and can be used for a wide range of applications beyond AI, including gaming, simulations, scientific computing, and more.
- Multiple Framework Support: Nvidia GPUs support multiple machine learning frameworks, including TensorFlow, PyTorch, and others, providing flexibility for diverse AI workloads.
- Mature Ecosystem:
- CUDA Platform: Nvidia’s CUDA programming model offers a well-established environment for developing and optimizing applications for GPUs. It has a rich set of libraries and tools that are widely adopted in the industry.
- Strong Performance:
- High Performance: Nvidia GPUs, particularly the latest models like the A100 and H100, offer exceptional performance for AI training and inference tasks, with advanced features such as tensor cores and large memory bandwidth.
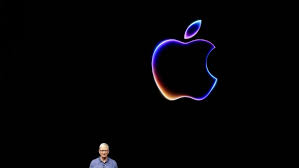
Disadvantages of Nvidia’s GPUs
- Cost:
- Higher Cost: High-performance Nvidia GPUs can be expensive, both in terms of initial investment and operational costs (e.g., power consumption and cooling).
- Energy Consumption:
- Power Usage: GPUs, especially those used for intensive AI tasks, can consume significant amounts of power, leading to higher energy costs compared to more specialized hardware like TPUs.
- Development Complexity:
- Complex Optimization: While CUDA provides powerful tools, optimizing applications for GPU architectures can be complex and may require specialized knowledge.
Broader Implications for the AI Hardware Market
- Diverse Solutions:
- Customization: The growing variety of AI hardware solutions—such as TPUs, custom silicon, and GPUs—reflects a trend towards more specialized and optimized computing solutions. This diversity allows organizations to choose the best hardware for their specific needs.
- Competition and Innovation:
- Driving Progress: Increased competition among hardware providers drives innovation and leads to continuous improvements in performance, efficiency, and capabilities.
- Market Dynamics:
- Adoption Trends: The adoption of different AI hardware solutions will vary based on factors such as performance requirements, cost considerations, and compatibility with existing tools and frameworks. This creates a dynamic market with opportunities for various players.
In summary, both Google’s TPUs and Nvidia’s GPUs have distinct advantages and disadvantages. TPUs offer specialized performance for TensorFlow-based tasks and can be cost-effective, while Nvidia GPUs provide versatility, a mature ecosystem, and strong performance across a broader range of applications. The choice between these options depends on specific needs, including the AI frameworks used, performance requirements, and cost considerations.
Features of Google’s TPUs
- Tensor Processing Units (TPUs):
- Purpose-Built for TensorFlow: TPUs are designed specifically to accelerate TensorFlow operations. They include specialized hardware for high-performance matrix operations, which are common in deep learning models.
- Matrix Multiply Units (MXUs): TPUs feature MXUs optimized for performing large-scale matrix multiplications, which are crucial for training and inference in neural networks.
- High Throughput and Efficiency:
- High Bandwidth: TPUs offer high memory bandwidth, enabling fast data transfers and computations, which is beneficial for handling large-scale AI models.
- Low Latency: The design of TPUs minimizes latency in executing deep learning operations, which helps speed up both training and inference processes.
- Energy Efficiency:
- Optimized Power Consumption: TPUs are designed to perform AI tasks with high energy efficiency, reducing power consumption compared to more general-purpose processors.
- Custom Hardware Design:
- Application-Specific Integrated Circuits (ASICs): TPUs are ASICs, meaning they are custom-designed for specific tasks. This specialization leads to enhanced performance for those tasks compared to general-purpose processors.
- Cloud Integration:
- Google Cloud Platform: TPUs are available through Google Cloud, making them accessible for cloud-based machine learning workloads. They integrate seamlessly with other Google Cloud services.
- Scalability:
- Cloud Scaling: TPUs can be easily scaled in Google Cloud to handle larger workloads by distributing computations across multiple units, providing flexibility for varying demands.
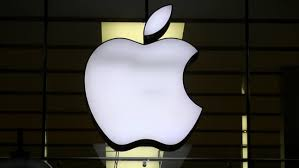
Features of Nvidia’s GPUs
- Graphics Processing Units (GPUs):
- Parallel Processing: Nvidia GPUs are designed to handle parallel processing tasks efficiently, which is ideal for AI and machine learning operations. They can perform many calculations simultaneously.
- CUDA Cores: Nvidia GPUs feature CUDA cores that accelerate various computing tasks, including those required for training and running AI models.
- Tensor Cores:
- AI Optimization: Recent Nvidia GPUs, such as those in the A100 and H100 series, include Tensor Cores specifically designed to accelerate tensor operations. These cores enhance performance for deep learning tasks, providing significant improvements in throughput.
- CUDA Platform:
- Programming Model: CUDA is a parallel computing platform and API model developed by Nvidia. It allows developers to leverage GPU acceleration and optimize their code for Nvidia hardware.
- Extensive Libraries: CUDA provides a range of libraries and tools, such as cuDNN (CUDA Deep Neural Network library) and TensorRT (a deep learning inference optimizer), which support various AI and machine learning tasks.
- Versatility:
- Broad Applications: Nvidia GPUs are versatile and can be used for a wide range of applications beyond AI, including gaming, simulation, and scientific computing. This makes them suitable for diverse workloads.
- Framework Support: Nvidia GPUs support a variety of machine learning frameworks, including TensorFlow, PyTorch, Keras, and more, providing flexibility for developers.
- High Performance and Large Memory:
- High Throughput: Nvidia GPUs offer high performance with substantial computational power, large memory bandwidth, and high core counts, which are crucial for handling complex AI models and large datasets.
- Large Memory: Modern Nvidia GPUs feature substantial amounts of high-speed memory (e.g., HBM2 or GDDR6), which is important for training large models and managing large datasets.
- Development Ecosystem:
- Software and Tools: Nvidia provides a comprehensive suite of software tools, including NVIDIA Nsight for debugging, profiling, and optimizing GPU-accelerated applications. The ecosystem also includes libraries like cuBLAS and cuFFT for optimized mathematical computations.
Comparison of Key Features
- Specialization vs. Versatility:
- TPUs: Specialized hardware tailored for TensorFlow and specific deep learning tasks. This specialization can lead to superior performance for these tasks but might lack versatility.
- GPUs: Versatile hardware suitable for a wide range of applications beyond AI, including graphics, simulation, and more. They provide flexibility in choosing different frameworks and applications.
- Performance Optimization:
- TPUs: Optimized for matrix operations and TensorFlow, providing high throughput and efficiency for specific AI tasks.
- GPUs: Offer strong performance with Tensor Cores for AI workloads, as well as parallel processing capabilities for a broad range of tasks.
- Energy Efficiency:
- TPUs: Generally designed to be more energy-efficient for AI tasks compared to GPUs.
- GPUs: While powerful, high-performance GPUs may consume more power, especially under intensive workloads.
- Availability and Integration:
- TPUs: Primarily available through Google Cloud, which integrates well with Google’s AI and cloud services.
- GPUs: Available from multiple vendors and can be used in a variety of environments, including on-premises systems and multiple cloud providers.
In summary, Google’s TPUs and Nvidia’s GPUs each have distinct features and strengths. TPUs are highly specialized for TensorFlow and deep learning, offering high performance and efficiency for these specific tasks. Nvidia’s GPUs provide versatile, high-performance computing suitable for a wide range of applications, with a robust development ecosystem supporting multiple frameworks and workloads.
Leave feedback about this