OpenAI is paving the way for a new era of AI as a service and increasing its model training materials.
It sounds like you’re referring to OpenAI’s new offerings related to GPT-4o and fine-tuning capabilities for companies. Fine-tuning is a powerful tool for businesses looking to adapt AI models like GPT-4o to better fit their specific needs and use cases. Here’s a brief overview of what this might entail:
- Custom Model Training: Companies can fine-tune GPT-4o with their own data to enhance performance in particular areas, whether it’s industry-specific jargon, company protocols, or customer service nuances.
- Improved Accuracy: Fine-tuning helps the model understand and generate more accurate responses relevant to a company’s specific context, leading to better performance and user satisfaction.
- Enhanced Personalization: Businesses can tailor the model’s behavior and responses to align with their brand voice and customer interactions.
- Use Case Flexibility: Whether for chatbots, content generation, or data analysis, fine-tuning allows businesses to customize the model to fit a wide range of applications.
- Integration and Support: OpenAI likely provides guidance and support for companies to seamlessly integrate and deploy their fine-tuned models. GPT
If you’re looking into this for a business or project, considering the scope of your needs and how you want the model to be adapted will help in making the most out of fine-tuning capabilities.
1. Fine-Tuning Process:
- Data Collection: Businesses start by gathering relevant data that reflects their specific use cases. This could include customer interactions, company-specific documents, industry jargon, or any other relevant information.
- Model Customization: The collected data is used to fine-tune the GPT-4o model. Fine-tuning adjusts the model’s parameters so that it better understands and generates content based on the specific data it’s trained on.
- Evaluation and Iteration: After fine-tuning, the model’s performance is evaluated through testing and feedback. Iterative adjustments may be made to ensure the model meets the desired standards of accuracy and relevance. GPT
2. Benefits for Businesses:
- Industry-Specific Knowledge: Fine-tuning can equip the model with deep insights into niche industries, enabling it to handle domain-specific queries with greater expertise.
- Enhanced Customer Experience: By tailoring the model to reflect the company’s tone and style, businesses can offer more personalized and consistent interactions with customers, improving satisfaction.
- Efficiency and Productivity: Custom models can streamline operations by automating responses and tasks that are highly specific to the business, reducing manual effort and increasing efficiency.
- Brand Consistency: Fine-tuned models help maintain a consistent brand voice across various customer touchpoints, reinforcing brand identity and messaging.
3. Use Cases:
- Customer Support: Fine-tuned models can handle customer service inquiries more effectively by understanding company-specific protocols and providing accurate, relevant responses. GPT
- Content Creation: Businesses can use fine-tuned models to generate marketing content, reports, or other written materials that align with their style and objectives.
- Data Analysis: Models can be fine-tuned to interpret and analyze data relevant to specific business needs, offering insights and recommendations tailored to the company’s context.
4. Integration and Deployment:
- Seamless Integration: Fine-tuned models can be integrated into existing systems and workflows, such as customer relationship management (CRM) tools, content management systems (CMS), and chat platforms.
- Support and Maintenance: OpenAI typically offers support to help with the deployment and ongoing maintenance of the fine-tuned models, ensuring they continue to perform well over time. GPT
5. Ethical and Privacy Considerations:
- Data Security: Ensuring that sensitive company data used for fine-tuning is handled securely and complies with privacy regulations is crucial.
- Bias Mitigation: It’s important to be aware of potential biases in the training data and take steps to mitigate them, ensuring the model operates fairly and ethically.
6. Future Developments: GPT
- Ongoing Improvements: As AI technology evolves, there may be new features and improvements in fine-tuning capabilities, offering businesses even more ways to customize and optimize their models.
- Advanced Features: Future updates might include more sophisticated tools for customization, allowing for even finer control over the model’s behavior and output.
1. Fine-Tuning Process
Data Collection and Preparation:
- Types of Data: Data used for fine-tuning can include text from emails, chat logs, documents, and other sources relevant to your business. The data should be clean, representative of your use case, and formatted properly.
- Data Privacy: It’s important to ensure that the data used complies with privacy regulations and does not contain sensitive information unless properly anonymized.
Fine-Tuning Procedure: GPT
- Initial Training: The model is first trained on general data, allowing it to learn a broad range of language patterns and information.
- Custom Training: Fine-tuning involves additional training on your specific dataset. This helps the model to specialize in the language and context relevant to your business.
- Hyperparameters: During fine-tuning, hyperparameters such as learning rate and batch size are adjusted to optimize the model’s performance on your data.
Evaluation and Iteration:
- Testing: After fine-tuning, the model is tested using separate validation data to assess its performance and accuracy. Common metrics include precision, recall, and F1 score.
- Feedback Loop: Based on testing results, further adjustments may be made. This iterative process helps in refining the model to better meet the desired outcomes.
2. Benefits for Businesses
Industry-Specific Knowledge: GPT
- Contextual Understanding: The fine-tuned model understands industry-specific terminology and concepts, improving its ability to respond accurately to specialized queries.
- Example: For a legal firm, the model could be fine-tuned with legal documents and case studies to provide accurate legal information.
Enhanced Customer Experience:
- Personalization: The model can generate responses that reflect the company’s brand voice and tone, making interactions feel more authentic and tailored.
- Consistency: Ensures uniformity in customer interactions, which helps in building trust and reliability.
Efficiency and Productivity:
- Automation: Automates routine tasks and responses, freeing up human resources for more complex and strategic activities.
- Scalability: Handles a large volume of interactions or content generation efficiently, scaling with business needs. GPT
Brand Consistency:
- Voice and Tone: Tailors the model to reflect specific branding guidelines and communication styles, ensuring consistent messaging across all platforms.

3. Use Cases
Customer Support:
- Automated Responses: Handles FAQs and common support queries, providing quick and accurate answers based on company-specific information.
- Case Management: Assists in categorizing and routing support tickets based on the content and urgency.
Content Creation:
- Marketing Materials: Generates blog posts, social media updates, and marketing copy aligned with the brand’s voice.
- Reports and Documentation: Produces business reports, product descriptions, and technical documentation. GPT
Data Analysis:
- Insight Extraction: Analyzes large volumes of text data to extract actionable insights and trends relevant to the business.
- Predictive Analytics: Helps in forecasting trends and customer behavior based on historical data.
4. Integration and Deployment
Integration:
- Systems Compatibility: The fine-tuned model can be integrated into various platforms such as customer support chatbots, CRM systems, and content management tools.
- API Access: Often, businesses interact with the model through APIs, allowing for seamless integration with existing software. GPT
Deployment:
- Scalability: Deployed in cloud environments to handle varying loads and ensure availability.
- Monitoring: Ongoing monitoring of the model’s performance to address any issues and ensure it meets the desired standards.
5. Ethical and Privacy Considerations
Data Security:
- Compliance: Ensure that data used for fine-tuning complies with GDPR, CCPA, or other relevant data protection regulations.
- Anonymization: Sensitive information should be anonymized or excluded to protect privacy.
Bias Mitigation:
- Diverse Data: Use diverse datasets to reduce biases and ensure the model represents various perspectives fairly. GPT
- Regular Audits: Periodically audit the model for biased outputs and adjust the training data as needed.
6. Future Developments
Advanced Features:
- Customizable Parameters: Future updates may offer more granular control over model parameters and behavior.
- Enhanced Tools: New tools for easier fine-tuning and model management could become available.
AI Evolution:
- Model Improvements: As AI technology advances, new versions of models may offer better performance, efficiency, and capabilities.
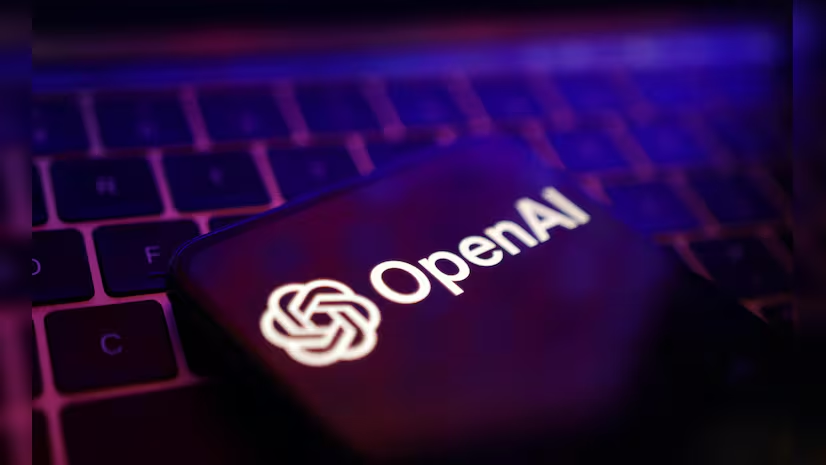
General Questions
1. What is GPT-4o? GPT-4o is an advanced language model developed by OpenAI, designed to generate human-like text based on the input it receives. It is the latest iteration in the GPT series, known for its high accuracy and versatility in various language tasks.
2. What does fine-tuning a model involve? Fine-tuning is the process of training a pre-existing model, like GPT-4o, on a specialized dataset to adapt it to specific tasks or domains. This helps the model perform better in contexts relevant to the business or use case. GPT
Fine-Tuning Process
3. How do I start the fine-tuning process?
- Data Preparation: Gather and prepare data that reflects your business needs.
- Training: Use the prepared data to train the model, adjusting hyperparameters as needed.
- Evaluation: Test the model’s performance and refine it based on feedback and results.
4. What kind of data is needed for fine-tuning? Data should be relevant to your specific use case, such as customer service interactions, industry-specific documents, or any other text that reflects the context in which the model will be used.
5. How long does fine-tuning take? The duration varies depending on the size of the dataset and the complexity of the fine-tuning task. It can range from a few hours to several days.
Benefits
6. How can fine-tuning benefit my business?
- Improved Accuracy: Tailors the model to provide more accurate and relevant responses.
- Enhanced Personalization: Aligns the model’s output with your brand’s voice and style.
- Increased Efficiency: Automates tasks and interactions, improving productivity. GPT
7. Can fine-tuning help with customer support? Yes, fine-tuning can improve customer support by enabling the model to handle queries with better understanding of your company’s specific products, services, and protocols.
Integration and Deployment
8. How is a fine-tuned model integrated into existing systems? Fine-tuned models are typically accessed via APIs, allowing integration with chatbots, CRM systems, and other software platforms used in your business.
9. What kind of support is available for deployment? OpenAI provides guidance and support for integrating and maintaining the fine-tuned model, including help with API usage and performance monitoring.
Ethics and Privacy
10. How is data privacy handled during fine-tuning? Data used for fine-tuning should be anonymized and handled according to privacy regulations like GDPR or CCPA. OpenAI ensures that sensitive information is managed securely.
11. What measures are in place to prevent bias in the fine-tuned model?
- Diverse Training Data: Incorporate diverse datasets to minimize bias.
- Regular Audits: Periodically review and adjust the model to address any biased outputs. GPT
Costs and Licensing
12. What are the costs associated with fine-tuning? Costs vary depending on factors such as dataset size, complexity of the fine-tuning task, and the scale of deployment. It’s best to consult with OpenAI for specific pricing details.
13. Are there licensing requirements for using a fine-tuned model? Licensing requirements depend on the usage and deployment of the fine-tuned model. Check OpenAI’s terms of service or contact their sales team for detailed licensing information.
Future Developments
14. Will there be updates to fine-tuning capabilities? Yes, as AI technology evolves, new features and improvements for fine-tuning may become available. OpenAI regularly updates its models and tools to incorporate the latest advancements.
15. How can I stay informed about new features and updates? Stay connected with OpenAI through their website, newsletters, and official communication channels for the latest news and updates on their offerings.
Advantages of Fine-Tuning GPT-4o
**1. Improved Relevance and Accuracy
- Customized Responses: Fine-tuning allows the model to generate responses that are more relevant and accurate for specific business contexts, such as industry jargon or company-specific procedures.
- Enhanced Performance: The model becomes better at understanding and addressing the unique needs and challenges of your business.
**2. Increased Personalization
- Brand Consistency: Tailors the model’s output to match your company’s voice and style, ensuring a consistent and authentic customer experience.
- User Experience: Provides personalized interactions that resonate more with your target audience. GPT
**3. Operational Efficiency
- Automation: Automates repetitive tasks such as answering FAQs, generating reports, or managing routine queries, freeing up human resources for more complex tasks.
- Scalability: Handles large volumes of interactions or content generation efficiently, adapting to business growth.
**4. Cost Savings
- Reduced Manual Work: Minimizes the need for manual intervention in routine tasks, potentially lowering operational costs.
- Efficient Resource Allocation: Allows human resources to focus on higher-value activities, improving overall productivity. GPT
**5. Enhanced Data Analysis
- Insights and Trends: Analyzes text data to provide actionable insights, identify trends, and make data-driven decisions tailored to your business needs.
- Predictive Capabilities: Offers predictive analytics based on historical data, aiding in strategic planning and forecasting.
**6. Flexibility and Adaptability
- Custom Use Cases: Supports a wide range of applications, from customer service to content creation, adaptable to various business needs and industries.
- Iterative Improvement: Allows for continuous improvement based on feedback and changing business requirements. GPT
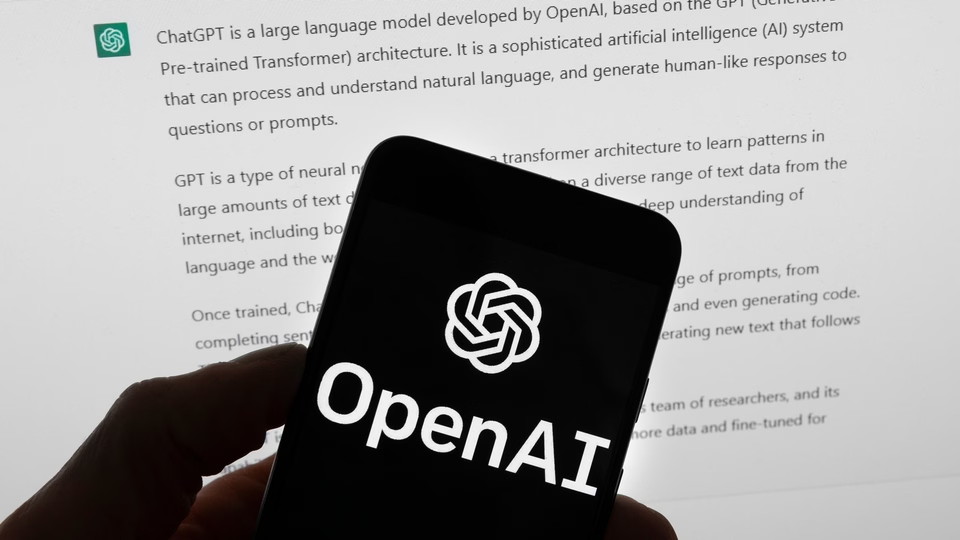
Disadvantages of Fine-Tuning GPT-4o
**1. Resource Intensive
- Data Preparation: Requires gathering, cleaning, and formatting relevant data, which can be time-consuming and resource-intensive.
- Computational Costs: Fine-tuning involves significant computational resources, which can be costly, especially for large datasets or complex tasks.
**2. Risk of Overfitting
- Specialization vs. Generalization: Fine-tuning can lead to overfitting, where the model becomes too specialized to the fine-tuning data and performs less effectively on general tasks or unexpected inputs.
**3. Data Privacy and Security
- Sensitive Information: Handling and processing sensitive or proprietary data for fine-tuning raises concerns about data privacy and security. Proper measures must be in place to protect this information.
- Compliance: Ensuring compliance with data protection regulations (e.g., GDPR, CCPA) can be complex and requires careful management. GPT
**4. Bias and Fairness
- Training Data Bias: If the fine-tuning data contains biases, the model may inherit and perpetuate these biases, potentially leading to unfair or discriminatory outcomes.
- Bias Mitigation: Requires ongoing monitoring and adjustment to address any biases that may emerge.
**5. Maintenance and Monitoring
- Ongoing Oversight: The fine-tuned model requires regular maintenance, including monitoring performance, updating data, and refining the model based on feedback.
- Model Drift: Over time, the model’s performance may degrade if it’s not updated to reflect changes in language use or business requirements.
**6. Initial Costs and Complexity
- Implementation: Setting up and fine-tuning the model involves initial costs and technical complexity, which may be challenging for businesses with limited AI expertise.
- Learning Curve: There may be a learning curve associated with understanding and effectively using the fine-tuned model.
Conclusion
Fine-tuning GPT-4o offers significant benefits in terms of relevance, personalization, and efficiency but also comes with challenges related to resources, data privacy, and model maintenance. Careful consideration of these factors will help you make an informed decision about whether fine-tuning is the right approach for your business needs.
Bottom Line:
Fine-tuning GPT-4o can significantly enhance the relevance, personalization, and efficiency of AI applications tailored to your business needs. It allows for improved accuracy, brand consistency, and operational automation, leading to better customer interactions and potential cost savings.
However, it requires careful management of resources, data privacy, and model biases. The process involves computational costs, potential for overfitting, and ongoing maintenance to ensure the model remains effective and fair.
In summary:
- Advantages: Customization, improved accuracy, personalized interactions, efficiency, cost savings, and enhanced data analysis.
- Disadvantages: Resource-intensive, risk of overfitting, data privacy concerns, potential for bias, and ongoing maintenance needs.
Evaluate these factors to determine if the benefits align with your business goals and if you’re prepared to manage the associated challenges effectively.
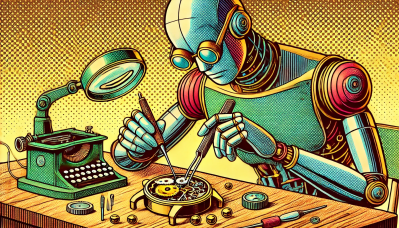
Core Benefits
- Enhanced Relevance and Precision:
- Custom Responses: Fine-tuning enables GPT-4o to generate highly relevant responses tailored to specific industry needs or business contexts.
- Accuracy: Improves the model’s understanding and output for specialized topics, resulting in fewer errors and more useful interactions.
- Personalized User Experience:
- Brand Voice: Aligns the model’s responses with your company’s tone and style, creating a consistent and engaging experience for users.
- Customer Engagement: Tailors interactions based on specific user preferences or business objectives, potentially increasing customer satisfaction and loyalty.
- Operational Efficiency and Scalability:
- Automation: Automates repetitive tasks and processes, such as handling customer inquiries or generating reports, which can reduce manual effort and operational costs.
- Scalable Solutions: Adapts to growing volumes of interactions or content needs, making it easier to scale your operations effectively.
- Cost-Effectiveness:
- Reduced Manual Work: By automating routine tasks, you can allocate human resources to more strategic or complex tasks, potentially lowering overall labor costs.
- Efficient Resource Use: Optimizes the use of computational resources and human effort, leading to better ROI for AI investments.
- Insightful Data Analysis:
- Actionable Insights: Provides deep insights and trends from your data, helping you make informed decisions based on the analysis of specialized content.
- Predictive Analytics: Uses historical data to predict future trends, aiding in strategic planning and forecasting.
Core Challenges
- Resource Demands:
- Data Preparation: Gathering, cleaning, and formatting data for fine-tuning can be resource-intensive and time-consuming.
- Computational Costs: The fine-tuning process requires substantial computational power, which can be costly, especially for large datasets or complex models.
- Risk of Overfitting:
- Specialization vs. Generalization: The model might become too tailored to the fine-tuning data, reducing its effectiveness for general use or novel queries.
- Performance Trade-offs: There’s a balance between achieving high performance on specific tasks and maintaining general adaptability.
- Data Privacy and Compliance:
- Sensitive Information: Handling and processing sensitive business or customer data requires stringent privacy measures and compliance with data protection regulations.
- Regulatory Compliance: Ensuring that data usage adheres to legal standards, such as GDPR or CCPA, is essential but can be complex.
- Bias and Fairness:
- Bias in Training Data: If the fine-tuning data contains biases, the model may produce biased outcomes, affecting fairness and inclusivity.
- Ongoing Monitoring: Requires continuous monitoring and adjustments to address any emerging biases or fairness issues.
- Maintenance and Management:
- Ongoing Updates: Regular maintenance and updates are necessary to keep the model effective and aligned with evolving business needs or language trends.
- Model Drift: Over time, the model’s performance may degrade if it’s not updated to reflect changes in language use or business requirements.
- Initial Costs and Complexity:
- Implementation Challenges: Setting up and fine-tuning the model involves initial costs and technical complexity, which may require expertise .